Filter for companies with Carbon (CO2) emissions.
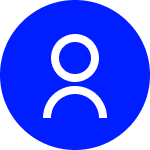
I wish to filter for U.S. companies with accessible CO2 emissions data. I have implemented this in the screener by establishing filters for, for instance, 5 and 10 years (filtering for GHG Scope 1 emissions > 0). Is there a method to filter for companies that possess emission data spanning the last 10 years (including those with values for the entire decade, as well as those with data available for less than 10 years)?
Is there also a way to obtain the data based on fiscal years beyond 2022, instead of FY 0?
thank you very much for the support
Best Answer
-
@quirin.braml thanks for your question. So I think one way of achieving this is to use a screener query to get a current universe and then go back in time to see what data is available. Also there are concepts such as Scope 1, Scope 2 and Scope 3 Emissions.
import refinitiv.data as rd
import numpy as
import pandas as pd
rd.open_session()Create a screener query for Americas:
df2 = rd.get_data(["SCREEN(U(IN(Equity(active,public,primary))/*UNV:Public*/), TR.HasESGCoverage==true, IN(TR.HeadquartersRegion,""Americas""), CURN=USD)"],["TR.CommonName;TR.HasESGCoverage;TR.HeadquartersRegion"])
Define a chunking function - so we remain compliant with API per call limits:
def chunks(l, n):
for i in range(0,len(l),n):
yield l[i:i+n]Create routine to loop through our chunks to get the data we need - i included some CO2 energy intensity calcs:
CEI = Employee Productivity * Energy Intensity * CO2 Intensity
rics1 = list(chunks(list(df2['Instrument'].values),1000))
rics1
maindf= pd.DataFrame()
for ric in rics1:
df3 = rd.get_data('0#.SPX',['TR.TRBCEconomicSector','TR.F.SalesPerEmp(Period=FY0)','TR.AnalyticEnergyUse(Period=FY0)','TR.CO2EmissionTotal(Period=FY0)','TR.CO2IndirectScope3(Period=FY0)','TR.EnergyUseTotal(Period=FY0)'])
df3.columns = ['Instrument','Sector','Revenue/Employee','EnergyUse/Revenue','CO2Emissions','CO2EmissionsScope3','EnergyUse']
df3['CO2Emissions/EnergyUse'] = df3['CO2Emissions'] / df3['EnergyUse']
df3['CO2Scope12/EnergyUse'] = df3['CO2Emissions'] / df3['EnergyUse']
df3['CO2Scope123/EnergyUse'] = (df3['CO2Emissions'] + df3['CO2EmissionsScope3']) / df3['EnergyUse']
df3['CEIScope12'] = df3['Revenue/Employee'] * df3['EnergyUse/Revenue'] * df3['CO2Emissions/EnergyUse']
df3['CEIScope123'] = d3['Revenue/Employee'] * df3['EnergyUse/Revenue'] * df3['CO2Scope123/EnergyUse']
if len(df3):
maindf = pd.concat([maindf, df3], axis=0)
maindfYou can then aggregate by sector and plot for example. I hope this can help.
0
Categories
- All Categories
- 6 AHS
- 36 Alpha
- 166 App Studio
- 6 Block Chain
- 4 Bot Platform
- 18 Connected Risk APIs
- 47 Data Fusion
- 33 Data Model Discovery
- 682 Datastream
- 1.4K DSS
- 613 Eikon COM
- 5.2K Eikon Data APIs
- 10 Electronic Trading
- Generic FIX
- 7 Local Bank Node API
- 3 Trading API
- 2.9K Elektron
- 1.4K EMA
- 248 ETA
- 552 WebSocket API
- 37 FX Venues
- 14 FX Market Data
- 1 FX Post Trade
- 1 FX Trading - Matching
- 12 FX Trading – RFQ Maker
- 5 Intelligent Tagging
- 2 Legal One
- 23 Messenger Bot
- 3 Messenger Side by Side
- 9 ONESOURCE
- 7 Indirect Tax
- 60 Open Calais
- 275 Open PermID
- 44 Entity Search
- 2 Org ID
- 1 PAM
- PAM - Logging
- 6 Product Insight
- Project Tracking
- ProView
- ProView Internal
- 22 RDMS
- 1.8K Refinitiv Data Platform
- 625 Refinitiv Data Platform Libraries
- 4 LSEG Due Diligence
- LSEG Due Diligence Portal API
- 4 Refinitiv Due Dilligence Centre
- Rose's Space
- 1.2K Screening
- 18 Qual-ID API
- 13 Screening Deployed
- 23 Screening Online
- 12 World-Check Customer Risk Screener
- 1K World-Check One
- 46 World-Check One Zero Footprint
- 45 Side by Side Integration API
- 2 Test Space
- 3 Thomson One Smart
- 10 TR Knowledge Graph
- 151 Transactions
- 143 REDI API
- 1.8K TREP APIs
- 4 CAT
- 26 DACS Station
- 121 Open DACS
- 1.1K RFA
- 104 UPA
- 191 TREP Infrastructure
- 228 TRKD
- 915 TRTH
- 5 Velocity Analytics
- 9 Wealth Management Web Services
- 83 Workspace SDK
- 11 Element Framework
- 5 Grid
- 18 World-Check Data File
- 1 Yield Book Analytics
- 46 中文论坛